AI Reduces Automotive Recalls: 2024 Guide
Discover how AI is reducing automotive recalls by integrating into manufacturing, maintenance, quality control, and software development processes. Learn about key benefits, challenges, and future trends in AI for the automotive industry.
Save 90% on your legal bills
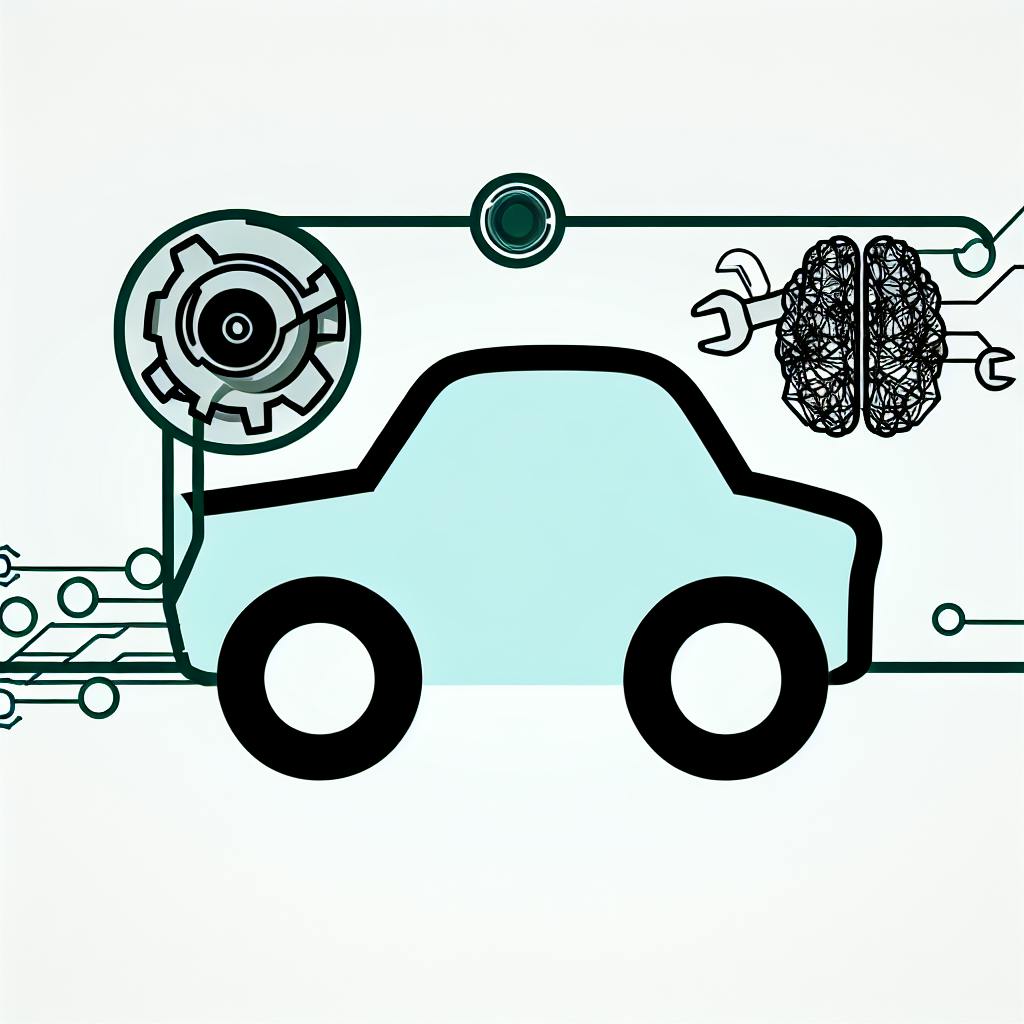
Artificial Intelligence (AI) is revolutionizing the automotive industry by dramatically reducing costly vehicle recalls. By integrating AI into manufacturing, maintenance, quality control, and software development processes, automakers can proactively identify and address potential defects before they become safety risks or compliance issues.
Key Benefits of AI for Reducing Recalls:
- Predictive Maintenance: AI analyzes sensor data to predict equipment failures, enabling proactive maintenance and preventing downtime.
- Enhanced Quality Control: AI vision systems automatically inspect vehicles with high accuracy, detecting even the smallest defects that humans may miss.
- Optimized Software Development: AI assists with software testing, defect prediction, and over-the-air updates, ensuring continuous improvement and rapid issue resolution.
Recommendations for Automakers:
- Develop an AI Strategy: Create a clear plan aligned with business goals, prioritizing areas like predictive maintenance, quality control, and software development.
- Build AI Skills: Invest in AI talent, infrastructure, and tools. Train employees and promote a data-driven culture.
- Focus on Data Management: Implement robust data governance, collection, and processing systems to ensure high-quality data for AI models.
- Collaborate: Work with AI providers, researchers, and industry partners to access expertise, share knowledge, and drive innovation.
- Use Responsible AI: Ensure AI systems are transparent, ethical, and compliant, prioritizing safety, privacy, and fairness.
By leveraging AI, the automotive industry can deliver safer, more reliable, and efficient vehicles, building consumer trust and driving growth.
Related video from YouTube
Understanding Car Recalls
Types of Car Recalls
There are several types of automotive recalls:
1. Safety Recalls
These are issued when a vehicle or component fails to meet minimum safety standards or has a defect that poses a safety risk. Safety recalls are the most common and serious type.
2. Emissions Recalls
Issued when a vehicle does not comply with federal emissions standards. These recalls aim to fix components that impact a vehicle's emissions.
3. Software Recalls
Automakers may issue recalls to update a vehicle's software and fix bugs, glitches, or cybersecurity vulnerabilities.
4. Technical Service Bulletins (TSBs)
These are not full recalls but notifications to dealerships about common issues. TSBs may lead to recalls if the problem is deemed safety-related.
Common Reasons for Recalls
Some of the most frequent reasons for automotive recalls include:
Reason | Description |
---|---|
Airbag Issues | Defective airbags that may not deploy properly or could injure occupants. |
Brake Problems | Faulty brake components like brake lines, rotors, or the anti-lock braking system. |
Steering Issues | Problems with the steering wheel, column, linkages, or power steering systems. |
Electrical Faults | Wiring issues, shorts, or malfunctions in electrical components like lights or sensors. |
Fuel System Leaks | Leaks that increase fire risks from the fuel lines, tanks, or pumps. |
Seat Belt Defects | Seat belts that may not latch or restrain occupants properly in a crash. |
Software Glitches | Bugs in vehicle software that create safety risks like stalling or loss of control. |
Recall Process and Difficulties
When a safety defect is identified, automakers must follow these key steps:
- Report to NHTSA: Manufacturers are required to notify NHTSA within five days of learning about a defect.
- Remedy Development: Automakers must develop a repair procedure and secure replacement parts.
- Owner Notification: Manufacturers must send recall notices by mail to all registered owners.
- Free Repairs: Vehicle owners can have the recall remedy performed at authorized dealerships at no cost.
However, automakers face challenges like:
- Securing replacement parts quickly
- Getting owners to bring in recalled vehicles
- High costs associated with large-scale recalls
AI in Car Manufacturing
AI Uses in Manufacturing
AI plays a key role throughout the car manufacturing process:
1. Design and Testing
AI analyzes data to optimize vehicle designs for factors like safety and fuel efficiency. AI simulations test designs before building prototypes.
2. Production and Assembly
Precise AI-enabled robots work on assembly lines, reducing errors and speeding up production. AI learns to adapt techniques for different vehicle models.
3. Quality Control
AI vision systems inspect vehicles with high accuracy, identifying even tiny defects in paint, components, welds, and assemblies that humans may miss.
4. Supply Chain Management
AI forecasts demand, manages inventory, coordinates logistics, and identifies potential supply chain issues.
Benefits of AI in Manufacturing
Using AI in automotive manufacturing offers several advantages:
Benefit | Description |
---|---|
Defect Detection | AI vision systems detect flaws more accurately than human inspectors, improving product quality. |
Predictive Maintenance | AI analyzes sensor data to anticipate equipment failures, enabling proactive maintenance and reducing downtime. |
Process Optimization | AI identifies inefficiencies and optimizes processes for improved productivity, cost savings, and resource utilization. |
Efficiency Improvements | Automated AI systems work tirelessly with consistent performance, increasing throughput and yield. |
Customization | AI facilitates mass customization by rapidly adapting production lines to meet varying customer preferences. |
Real Examples
Leading automakers are using AI in their manufacturing operations:
- Tesla: AI for visual inspection, predictive maintenance, and process optimization across factories.
- General Motors: AI for quality control, assembly line optimization, and supply chain management.
- Ford: AI generates test cases for infotainment systems, reducing manual effort and time.
- BMW: AI for defect detection, predictive maintenance, and logistics optimization in production facilities.
- Toyota: AI for assembly lines, quality control, and supply chain management for improved efficiency and quality.
Predicting Equipment Issues with AI
What is Predictive Maintenance?
Predictive maintenance uses data and AI to identify potential equipment failures before they happen. Instead of waiting for problems or following fixed maintenance schedules, it monitors real-time conditions to catch issues early. This approach prevents costly downtime and extends equipment lifespan.
How AI Helps Predict Maintenance Needs
AI techniques like machine learning analyze data to find patterns that indicate potential failures. For example, deep learning models can process complex data like vibration signals to detect anomalies that may signal issues.
Data Sources for Predictive Maintenance
Predictive maintenance relies on various data, including:
- Sensor data: Real-time readings on temperature, vibration, pressure, etc.
- Maintenance records: Past repairs, replacements, and maintenance activities.
- Operational data: Usage patterns, environmental conditions, and other performance factors.
Combining these data sources gives AI algorithms a comprehensive view to accurately identify potential failures.
Benefits Over Regular Maintenance
Regular Maintenance | Predictive Maintenance with AI |
---|---|
Reactive - addresses issues after they occur | Proactive - predicts and prevents failures |
Follows fixed schedules, regardless of condition | Maintenance based on real-time condition monitoring |
Increased downtime due to unexpected breakdowns | Reduced downtime by addressing issues before failures |
Higher costs for unplanned repairs | Lower costs by optimizing maintenance schedules |
Shorter equipment lifespan due to unaddressed issues | Extended lifespan by addressing issues early |
Limited data for decision-making | Data-driven insights for optimized maintenance planning |
AI for Quality Checks and Inspections
Automated Visual Inspection
AI-powered visual inspection systems use computer vision and deep learning to automate quality control in car manufacturing. These systems capture high-resolution images of vehicle parts and assemblies, which are analyzed by AI models trained to identify defects and irregularities.
The AI models, based on convolutional neural networks (CNNs), are trained on large datasets of labeled images. This allows them to recognize patterns and detect even the smallest imperfections that humans may miss. This automated inspection process ensures consistency and accuracy, reducing the risk of defective parts reaching final vehicle assembly.
Finding and Classifying Defects
AI models can not only detect defects but also classify them into categories like scratches, dents, paint flaws, or misalignments. This classification helps manufacturers identify the root causes of defects and implement targeted corrective actions.
For example, an AI system may detect and classify a dent on a body panel, providing details like its location, size, and severity. This level of detail enables efficient rework or replacement of the affected component, minimizing the need for extensive disassembly or rework.
Integrating AI into Quality Control
AI-based visual inspection systems are designed to seamlessly integrate into existing quality control workflows. They can be deployed at various stages of the manufacturing process, from component inspection to final vehicle checks.
The inspection data generated by these systems is typically fed into centralized quality management platforms, where it can be analyzed, tracked, and shared across different departments and facilities. This integration allows for real-time monitoring of quality issues, enabling prompt corrective actions and facilitating continuous improvement initiatives.
Comparison
Traditional Quality Control | AI-based Quality Control |
---|---|
Manual inspections prone to human error | Automated and consistent inspections |
Limited inspection capacity and speed | High-speed inspections with high throughput |
Difficulty detecting small or complex defects | Can detect even minute defects |
Subjective and inconsistent defect classification | Objective and consistent defect classification |
Reliance on skilled inspectors | Scalable to changing requirements |
Limited data collection and analysis | Comprehensive data collection and analysis |
Reactive approach to quality issues | Proactive identification and prevention of quality issues |
sbb-itb-ea3f94f
AI in Car Software Development
Challenges in Car Software
1. Complex Systems: Modern cars have many software parts that control different systems, like infotainment and driver assistance. Integrating and ensuring these systems work together is difficult.
2. Safety Rules: Car software must follow strict safety standards and regulations to ensure critical functions are reliable and secure. Thorough testing and validation are required, adding complexity.
3. Compatibility Issues: With multiple software modules from different suppliers, ensuring seamless communication and data exchange between these systems is challenging. Standardization and robust integration testing are essential.
AI for Software Testing
AI and machine learning can improve the efficiency and accuracy of software testing in the auto industry:
- Automated Test Cases: AI algorithms can analyze software requirements and automatically generate comprehensive test cases, reducing the time and effort required for manual test case creation.
- Defect Prediction and Detection: By analyzing historical data and code patterns, AI models can predict potential defects and identify high-risk areas, enabling targeted testing efforts.
- Test Optimization: AI can optimize test suite execution by prioritizing test cases based on their likelihood of detecting defects, reducing overall testing time and costs.
- Regression Testing: AI-powered regression testing can identify and prioritize tests that need to be re-executed after code changes, ensuring efficient testing of modified functionality.
Over-the-Air Updates
AI plays a crucial role in enabling over-the-air (OTA) software updates for vehicles:
- Monitoring and Analysis: AI systems can continuously monitor vehicle data and software performance, identifying issues or areas that require updates.
- Update Prioritization: AI algorithms can prioritize software updates based on factors such as severity, impact, and vehicle usage patterns, ensuring critical updates are delivered promptly.
- Validation and Testing: Before deploying updates, AI can assist in validating and testing the software changes, reducing the risk of introducing new issues.
- Targeted Updates: AI can facilitate targeted updates, delivering software changes only to the specific vehicle models or configurations that require them, optimizing bandwidth usage and minimizing unnecessary updates.
Cybersecurity and Safety with AI
AI techniques are increasingly being used to enhance cybersecurity and safety in automotive software:
- Threat Detection: AI models can analyze vehicle data and network traffic patterns to detect anomalies and potential cyber threats in real-time.
- Vulnerability Identification: AI algorithms can scan software code and identify vulnerabilities or weaknesses that could be exploited by cybercriminals.
- Predictive Maintenance: By analyzing sensor data and vehicle usage patterns, AI can predict potential component failures or safety issues, enabling proactive maintenance and preventing recalls.
- Autonomous Driving Safety: AI is essential for enabling safe autonomous driving, with algorithms for object detection, path planning, and decision-making based on real-time data analysis.
Traditional Software Development | AI-Assisted Software Development |
---|---|
Manual test case creation | Automated test case generation |
Limited defect detection | Predictive defect detection and prevention |
Fixed testing schedules | Optimized testing based on risk and priority |
Inefficient regression testing | Targeted regression testing for code changes |
Limited monitoring and analysis | Continuous monitoring and analysis |
Delayed software updates | Prioritized and targeted software updates |
Reactive cybersecurity measures | Proactive threat detection and vulnerability identification |
Limited predictive maintenance | AI-enabled predictive maintenance |
Reliance on human decision-making | AI-powered decision-making for autonomous driving |
Regulations and Ethics
Current and Upcoming Rules
As AI becomes more common in cars, new rules are being made to ensure it is used safely and properly. The UN Regulation on Cybersecurity (Doc 11) provides guidelines for identifying and managing cybersecurity risks, verifying risk assessments, and monitoring cyber-attacks. Manufacturers must show they follow these requirements before selling vehicles.
Additionally, governments and regulatory bodies are working on establishing specific AI regulations for automobiles (Doc 10). While there are limited rules currently, there is a growing need for a comprehensive global framework to govern the use of AI in vehicles and ensure consistent standards across regions.
Ethical Concerns
The use of AI in automobiles raises several ethical concerns that must be addressed:
Data Privacy: As AI systems collect and process large amounts of data, including potentially personal information, it is crucial to implement robust data privacy measures to protect user privacy and prevent unauthorized access or misuse of data (Doc 12).
Avoiding Bias: AI algorithms must be designed and trained to avoid biases that could lead to unfair or discriminatory decision-making (Doc 4). Transparency and fairness in AI systems are essential to ensure ethical and equitable outcomes.
Transparency and Accountability: AI systems should be transparent in their decision-making processes, and there should be clear guidelines and regulations in place to hold individuals and companies accountable for any ethical breaches related to AI in automobiles (Doc 12).
Safety and Reliability: The safety and reliability of AI-driven systems, particularly in autonomous driving, are paramount. Rigorous testing, validation, and continuous monitoring are necessary to ensure AI systems operate as intended and do not pose risks to human life (Doc 9).
Best Practices for Responsible AI Use
To address ethical concerns and promote responsible AI use in the automotive industry, the following best practices should be adopted:
- Ethical Framework and Guidelines: Develop a comprehensive ethical framework and guidelines for AI development and deployment, addressing issues such as data privacy, avoiding bias, transparency, and accountability (Doc 10).
- Collaboration and Standardization: Foster industry-wide collaboration and establish standardized practices for the ethical use of AI in automobiles. This includes sharing threat intelligence, best practices, and mitigation techniques (Doc 6).
- Human Oversight and Control: Ensure that AI systems in automobiles operate under appropriate human oversight and control, particularly in critical decision-making scenarios (Doc 4).
- Continuous Monitoring and Improvement: Implement processes for continuous monitoring and improvement of AI systems, addressing emerging threats, vulnerabilities, and ethical concerns as they arise (Doc 6).
- Responsible AI Principles: Incorporate responsible AI principles, such as human-centered design, fairness, transparency, and privacy protection, into the development and deployment of AI systems in automobiles (Doc 1).
Ethical Concern | Best Practice |
---|---|
Data Privacy | Implement robust data privacy measures |
Avoiding Bias | Design and train AI algorithms to avoid biases |
Transparency and Accountability | Establish clear guidelines and regulations for accountability |
Safety and Reliability | Rigorous testing, validation, and continuous monitoring |
Reducing Recalls with AI
Adopting AI Strategies
To effectively use AI in manufacturing and reduce recalls, a systematic approach is key:
1. Assess Current Processes
Thoroughly analyze existing manufacturing processes, quality control measures, and data collection methods to identify areas where AI can help.
2. Develop an AI Plan
Create a plan outlining the implementation of AI solutions, including timelines, milestones, and measurable goals for reducing recalls.
3. Establish Data Infrastructure
Implement robust data management systems to collect, store, and process the vast data required for AI algorithms. Integrate data from various sources like sensors, quality checks, and maintenance records.
4. Implement AI Solutions
Deploy AI-powered predictive maintenance, quality inspection, and software testing solutions tailored to your manufacturing processes. Continuously monitor and refine these systems based on real-world performance.
5. Train Employees
Invest in training programs to equip employees with the knowledge and skills to work effectively with AI systems.
Managing Data for AI
Effective data management is crucial for successful AI implementation:
- Data Collection and Integration: Establish mechanisms to collect and integrate data from various sources while ensuring data quality and consistency.
- Data Storage and Processing: Implement scalable and secure data storage solutions with sufficient computing power to process and analyze large volumes of data.
- Data Governance: Develop and enforce data governance policies to ensure data privacy, security, and regulatory compliance.
- Data Labeling and Annotation: Invest in data labeling and annotation processes to prepare high-quality training datasets for AI algorithms.
Challenges in Adopting AI
While AI offers significant benefits in reducing recalls, its adoption in the automotive industry faces several challenges:
Challenge | Description |
---|---|
Cost | Implementing AI solutions can be capital-intensive, requiring investments in hardware, software, data infrastructure, and skilled personnel. |
Skills Gap | There is a shortage of professionals with expertise in AI, machine learning, and data science, making it challenging to build and maintain AI systems. |
Organizational Resistance | Overcoming resistance to change and fostering a culture that embraces AI and data-driven decision-making can be difficult. |
To overcome these challenges, automotive companies should:
- Develop a comprehensive AI strategy aligned with business goals and allocate appropriate resources.
- Collaborate with academic institutions, research organizations, and technology partners to access talent and expertise.
- Provide comprehensive training and change management programs to upskill employees and promote AI adoption.
Success Stories
Several automotive companies have successfully implemented AI to reduce recalls and improve quality:
- Tesla: Leverages AI for predictive maintenance, software updates, and quality inspections, significantly reducing recalls and improving customer satisfaction.
- Ford: Utilizes AI-powered visual inspection systems to detect defects during manufacturing, resulting in a 90% reduction in recall rates for certain components.
- BMW: Employs AI algorithms to analyze sensor data and predict component failures, enabling proactive maintenance and reducing unplanned downtime.
These success stories demonstrate the potential of AI in reducing recalls and highlight the importance of a strategic and comprehensive approach to AI adoption in the automotive industry.
Future of AI in Cars
New AI Tech
New AI tech will change how cars are made. For example, AI models like ChatGPT can help with software coding, testing, and fixing bugs. This speeds up getting new software to market. Better computer vision will improve finding defects and checking quality. Reinforcement learning algorithms can optimize manufacturing and maintenance.
Self-Driving and Connected Cars
AI powers self-driving and connected cars. Deep learning models use data from sensors and cameras to understand the surroundings, plan routes, and control the vehicle. AI also enables car-to-car and car-to-infrastructure communication, improving safety and traffic flow. As these technologies improve, AI will reduce recalls by finding issues early and enabling over-the-air software updates.
Industry Teamwork
Car companies are partnering with AI providers and researchers to speed up innovation and use cutting-edge AI solutions. These partnerships share knowledge, access talent and expertise, and develop industry-specific AI models and tools. Working together helps address challenges like data quality, algorithm reliability, and regulations.
Recommendations for Automakers
To stay competitive and use AI effectively, car companies should:
- Develop an AI Plan: Create a clear AI strategy aligned with business goals, prioritizing areas like predictive maintenance, quality control, and software development.
- Build AI Skills: Invest in AI talent, infrastructure, and tools. Train existing employees and promote a data-driven culture.
- Focus on Data Management: Implement robust data governance, collection, and processing systems to ensure high-quality data for AI models.
- Collaborate: Work with AI providers, researchers, and industry partners to access expertise, share knowledge, and drive innovation.
- Use Responsible AI: Ensure AI systems are transparent, ethical, and comply with regulations, prioritizing safety, privacy, and fairness.
New AI Tech | Benefits |
---|---|
AI models like ChatGPT | Assist with software development, testing, and debugging |
Computer vision advancements | Enhance defect detection and quality control |
Reinforcement learning algorithms | Optimize manufacturing processes and predictive maintenance |
AI in Connected Cars | Applications |
---|---|
Deep learning models | Perceive environment, plan routes, control vehicle |
Vehicle-to-Vehicle (V2V) communication | Enhance safety and traffic management |
Vehicle-to-Infrastructure (V2I) communication | Improve safety and traffic flow |
Over-the-air software updates | Proactively identify and address potential issues |
Conclusion
The car industry is quickly using AI to improve vehicle safety, quality, and efficiency. By putting AI into manufacturing, maintenance, quality control, and software development, automakers can greatly reduce recalls and build consumer trust.
AI systems can find defects early, optimize workflows, and enable proactive maintenance, preventing issues before they become costly recalls. AI also helps with software testing and over-the-air updates, allowing for continuous improvement and quick fixes.
As new AI technologies like advanced computer vision, AI models, and reinforcement learning emerge, the car industry will benefit from more automation, better quality control, and optimized processes. Working with AI providers and researchers will drive innovation and adoption of cutting-edge solutions.
To stay competitive and get the most from AI, automakers must:
- Develop AI strategies
- Invest in AI skills and infrastructure
- Prioritize data management
- Partner with others in the industry
- Use ethical and responsible AI practices
By using AI, the car industry can deliver safer, more reliable, and efficient vehicles, building consumer trust and driving growth.
Benefits of AI in the Automotive Industry
AI Application | Benefits |
---|---|
Manufacturing | Detect defects early, optimize workflows |
Predictive Maintenance | Prevent issues before costly recalls |
Quality Control | Enhance defect detection and quality assurance |
Software Development | Enable continuous improvement and rapid issue resolution |
Recommendations for Automakers
-
Develop an AI Plan
- Create a clear strategy aligned with business goals
- Prioritize areas like predictive maintenance, quality control, and software development
-
Build AI Skills
- Invest in AI talent, infrastructure, and tools
- Train employees and promote a data-driven culture
-
Focus on Data Management
- Implement robust data governance, collection, and processing systems
- Ensure high-quality data for AI models
-
Collaborate
- Work with AI providers, researchers, and industry partners
- Access expertise, share knowledge, and drive innovation
-
Use Responsible AI
- Ensure AI systems are transparent, ethical, and compliant
- Prioritize safety, privacy, and fairness