AI Legal Tech Regulation: Challenges & Right to Explanation
Explore the challenges and perspectives on regulating AI in legal technology, focusing on the right to explanation. Learn about emerging trends, recommendations, and key issues in the AI legal tech landscape.
Save 90% on your legal bills
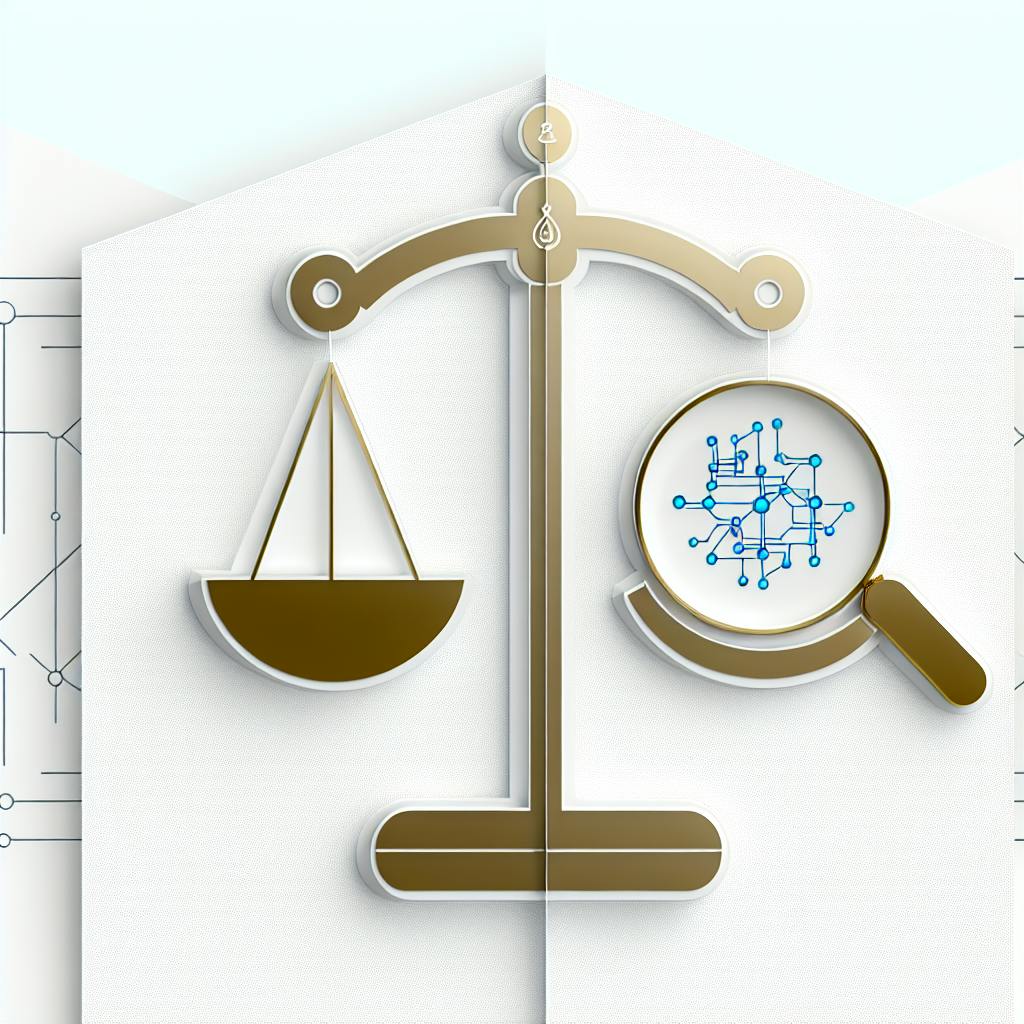
Regulating AI in legal technology is a growing concern as AI systems become more prevalent. A key issue is the "right to explanation" - the need for people to understand how AI makes decisions that affect them. This article explores the challenges of this right, recent regulations, and industry perspectives on making AI in legal tech transparent and accountable.
Related video from YouTube
Key Challenges
- Technical Difficulties: Explaining complex "black box" AI models and their decision-making processes
- Ethical Concerns: Addressing potential biases and ensuring fairness in AI legal tech systems
- Legal Challenges: Balancing transparency requirements like the GDPR's right to explanation with protecting intellectual property (IP) and trade secrets
- Compliance: Meeting evolving regulations like the proposed EU AI Act that set standards for AI transparency and accountability
Industry Perspectives
Stakeholder | View |
---|---|
Legal Experts | Emphasize the need for clear, understandable AI systems while balancing transparency and IP protection |
Technologists | Admit explaining "black box" AI is difficult, but are developing better tools for explainability |
Regulators | Pushing for clear standards on AI explainability, like the GDPR's right to explanation and the proposed EU AI Act |
Businesses | Recognize the importance of transparency for trust and ethics, but worry about revealing trade secrets |
Emerging Trends
- Explainable AI (XAI): Techniques like LIME, SHAP, and causal reasoning to make AI decision-making more interpretable
- New Regulations: Frameworks like the EU AI Act that categorize AI systems by risk and set transparency requirements
- Successful Implementations: Examples of organizations using XAI to build trust, like Healthily's AI Explainability Statement
- Privacy & Security Impact: Balancing transparency with protecting sensitive data and IP using methods like differential privacy
Recommendations
- Create an AI Explainability Statement detailing the system's purpose, data, decision process, fairness measures, and limitations
- Implement ethical AI governance with clear principles, oversight, monitoring, and accountability processes
- Balance innovation and compliance by staying updated on regulations, using flexible AI architectures, and investing in XAI tools
- Provide training on AI basics, transparency principles, regulations, and ethical considerations
- Foster collaboration between stakeholders to develop industry standards and best practices for AI explainability
sbb-itb-ea3f94f
Key Issues and Obstacles
Technical Difficulties in Explaining AI
AI models, especially deep learning ones, are often called "Black Boxes" because their decision-making process is hard to understand. These systems use complex math and large amounts of data, making it tough to explain their decisions in simple terms.
Ethical Concerns
AI in legal tech can have biases. If the training data has biases, the AI might make unfair decisions that affect certain groups more than others. Transparency helps spot and fix these biases, keeping the legal system fair.
Legal Challenges
The GDPR's Article 22 gives people the right to know how automated decisions that affect them are made. But the GDPR doesn't say how detailed these explanations need to be, causing confusion. Balancing this transparency with protecting intellectual property is tricky.
Balancing Transparency and IP Protection
Explaining an AI system's decisions might mean revealing sensitive details about its design or data, which could expose trade secrets. Legal tech companies need to find a way to be transparent without giving away their proprietary information.
Compliance with New Regulations
New rules are coming out to manage AI in legal tech, like the proposed EU AI Act, which sets standards for transparency. Companies need to follow these rules while still making effective AI tools.
Expert Views and Industry Insights
Legal Experts on AI Transparency
Legal experts stress the need for clear and understandable AI systems in legal tech. Chanley T. Howell, a partner at Foley & Lardner LLP, states, "Regulatory authorities often require companies to provide clear and transparent explanations for their actions and decisions related to using AI to meet compliance requirements, especially in the financial services industry. Failure to provide such explanations can lead to hefty fines and reputational damage."
Experts also point out the challenge of balancing transparency with protecting intellectual property. One legal scholar notes, "Providing information in a concise, transparent, intelligible, and easily accessible format, using clear and plain language is another hurdle to overcome. Technical information about the source code of the AI-system will certainly not be enough for the average data subject to comprehend, and is even protected against unlawful disclosure under IP-law."
Technologists' Perspective
AI developers admit that making AI models easy to understand is tough, especially for complex systems known as "Black Boxes." However, they are working on better tools to explain how AI makes decisions.
The Zendesk Customer Experience Trends Report 2024 states, "These expectations include developing better tools to help explain complex AI models so users can understand the AI decision-making process, which will help to increase trust and usability."
Regulators' Stance
Regulators are pushing for clear standards on AI explainability. The GDPR in the EU gives people the right to know how automated decisions, including those made by AI, affect them.
The proposed EU Artificial Intelligence Act also aims to regulate AI development with a focus on transparency and accountability. The article notes, "These regulations can standardize the use and development of AI, locally and globally. AI systems can be consistently more clear and trustworthy by emphasizing transparency, ethical considerations, and accountability."
Business Impact
Business leaders see the need for transparency in AI to build trust and ensure ethical use. However, they worry about revealing trade secrets.
The article highlights, "Explaining an AI system's decisions might mean revealing sensitive details about its design or data, which could expose trade secrets. Legal tech companies need to find a way to be transparent without giving away their proprietary information."
Businesses are looking for ways to balance being open about their AI systems while protecting their competitive edge and following new rules.
Emerging Trends and Developments
Advances in Explainable AI
Researchers are working on Explainable AI (XAI) to make AI systems easier to understand. Techniques like LIME (Local Interpretable Model-Agnostic Explanations) and SHAP (SHapley Additive exPlanations) help show how AI models make decisions by highlighting key features. Causal reasoning is another area that looks at the cause-and-effect relationships in AI decision-making.
New Regulatory Approaches
Governments are creating new rules to ensure AI transparency and accountability. The European Union's AI Act uses a risk-based approach, categorizing AI systems by their potential risks and setting different requirements for transparency and oversight. China's Interim Measures for Generative AI Services also provide guidelines for explainability and risk assessment.
Successful Implementation Examples
Some organizations have successfully used explainable AI to build trust. For example:
- Healthily: Published an AI Explainability Statement to show how its AI models work and ensure fairness.
- Zendesk: Offers insights into how its AI tools make decisions and provides resources to help users understand AI in customer service.
Privacy and Security Impact
Increasing AI transparency can raise privacy and security concerns. Revealing too much about an AI system could expose sensitive data or trade secrets. Companies need to balance providing explanations with protecting critical information. Privacy-enhancing technologies like differential privacy and secure multi-party computation can help maintain data privacy while ensuring transparency.
Future Directions
As AI becomes more common, the need for explainable and trustworthy AI systems will grow. Regulatory bodies may introduce more consistent standards for AI transparency. Advances in XAI techniques and user-friendly tools will make it easier for organizations to explain AI decision-making processes, building greater trust and accountability.
Recommendations and Best Practices
Creating an AI Explainability Statement
Organizations should publish an AI Explainability Statement to increase transparency. This statement should include:
- The purpose and use of the AI system
- Data sources and types used for training
- The decision-making process and key factors
- Steps to reduce bias and ensure fairness
- Limitations and risks of the AI system
- Human oversight and accountability processes
This transparency builds trust and shows a commitment to responsible AI use.
Ethical AI Governance
Setting up strong ethical AI governance is key for transparency and accountability. Important elements include:
- Clear principles and guidelines for AI use, aligned with industry standards
- Oversight committees with diverse members
- Continuous monitoring, risk assessment, and auditing of AI systems
- Ways to address issues like bias or privacy violations
- Training programs on ethical AI practices
Ethical AI governance helps identify and reduce risks while promoting responsible innovation.
Balancing Innovation and Compliance
Businesses need to balance innovation with regulatory compliance. Strategies include:
- Staying updated on new regulations and best practices
- Working with legal and compliance teams early in AI development
- Implementing strong testing and validation processes
- Using flexible AI architectures to meet changing requirements
- Investing in explainable AI tools to improve transparency
A proactive approach helps leverage AI's potential while minimizing risks.
Training and Education
Providing training for legal and tech teams is essential for explainable AI. Training should cover:
- Basics of AI and machine learning
- Principles of transparency and explainability
- Regulatory requirements and industry standards
- Best practices for developing explainable AI systems
- Ethical considerations and bias reduction techniques
Ongoing training helps teams navigate AI legal tech complexities and ensure compliance.
Stakeholder Collaboration
Collaboration between businesses, regulators, academics, and others is crucial for developing standards and best practices for AI explainability. Areas of collaboration include:
- Developing industry-specific guidelines
- Sharing case studies and lessons learned
- Conducting joint research on explainable AI techniques
- Participating in public-private partnerships
- Providing feedback on proposed regulations and policies
Working together helps create robust standards for AI transparency and accountability.
Conclusion
The rapid growth of AI in legal technology brings both opportunities and challenges. As AI systems become more advanced, the need for transparency, accountability, and ethical governance becomes more important. Ensuring people understand how AI makes decisions that affect them is a shared responsibility among legal professionals, technologists, regulators, and society.
Collaboration and open dialogue between stakeholders are key to developing frameworks and best practices that balance innovation with responsible AI use. By working together, we can use AI to improve the legal system while protecting individual rights.
To navigate this evolving landscape, organizations must stay informed about new regulations, invest in explainable AI techniques, and prioritize ethical AI governance. This approach helps leverage AI's capabilities while reducing risks and building public trust.
The path forward is clear: prioritize transparency, embrace collaboration, and uphold the core values of the legal profession. Through collective efforts, we can shape a future where AI improves the delivery of justice and the rule of law.
FAQs
What is the right to an explanation in AI?
The right to an explanation in AI means that organizations must provide clear explanations for decisions made by their AI systems, especially when these decisions impact individuals. This is to ensure transparency and accountability.
Under the European Union's General Data Protection Regulation (GDPR), people can ask for an explanation of automated decisions that affect them. This includes decisions made by AI systems using personal data.
This right is important because many AI systems, especially those using machine learning, are like "black boxes" – their decision-making processes are hard to understand. Providing explanations helps people see how and why decisions were made, so they can judge if the AI system is fair and accurate.
Implementing this right can be challenging because it requires AI systems to be understandable or to generate explanations that people can easily grasp. However, it is a key step in building trust and ensuring responsible use of AI in areas like legal technology.